Learning
What is RAG AI?
Find out how RAG AI integrates business data to enhance AI's response specificity and reliability.
%20copy.jpg)
Retrieval-Augmented Generation AI, or RAG AI for short, makes AI models better at providing accurate answers by using external information. Unlike traditional models, RAG AI integrates live data to enhance response specificity and reliability. In this article, we will explore what is RAG AI, its workings, benefits, and uses.
Key Takeaways
- Retrieval-Augmented Generation (RAG) enhances generative AI by integrating real-time data from external sources, improving accuracy and relevance of responses.
- RAG systems utilize vector databases for efficient information retrieval, allowing seamless access to both structured and unstructured data, thereby enriching response context.
- Despite its benefits, implementing RAG poses challenges such as high computational costs and data privacy concerns, necessitating robust governance frameworks.
What is Retrieval-Augmented Generation (RAG)?
The technique of retrieval-augmented generation (RAG) is designed to improve the precision and timeliness of AI results by utilizing external sources for additional knowledge. RAG’s core objective lies in embedding pertinent data from such resources into generative AI systems, thus elevating the dependability and relevance of content produced by these models. This enhancement addresses a crucial limitation observed in several conventional approaches.
Whereas typical generative AI models rely on static datasets that may become obsolete, the incorporation of both retrieval mechanisms and generative components permits access to contemporary information stored in databases. By forming this active linkage, it ensures that responses generated are relevant and compliant, since data used by AI comes from information stored in a managed database.
Facebook AI Research underscores one notable advantage of RAG: its capacity for fetching live updates from vast repositories thereby resolving issues associated with static intelligence seen among extensive language models. Such real-time integration substantially increases response accuracy, rendering these interactions more valuable to end-users.
By fusing both retrieval processes and generation techniques within their framework, RAG AI Models manage to provide solutions rich in contextual understanding as well as factual correctness. Their advanced capabilities offer enhanced potentialities over prior iterations of generative AIs which require accurate up-to-the-minute details. This positions them at the forefront for tasks necessitating high-precision informational output, for example, in customers service processes or law firms.
How Retrieval-Augmented Generation Works
Vector databases play an integral role in the RAG (Retrieval-Augmented Generation) system by housing numerical representations of data. This arrangement allows for quick and precise retrieval of pertinent information from these databases using vectorized user queries, significantly enhancing the capability to produce detailed responses.
At its essence, RAG harnesses a variety of external data sources through vector databases to boost the accuracy and relevance of AI-generated answers. The transformation of user inquiries into vectors facilitates this process, enabling the search within a vector database for germane information that can be used to enrich content with accurate context.
Utilizing both retrieval mechanisms and generative models, RAG systems are adept at offering up-to-date responses that integrate recent and related data — distinguishing them from conventional generation-only frameworks. In scenarios where precision is essential, like providing current financial statistics or stock prices in volatile markets, such hybrid systems are especially valuable for yielding results that reflect real-time conditions accurately.
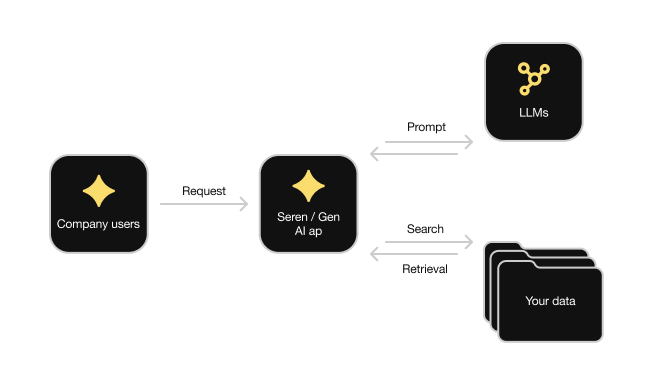
The Role of Vector Databases in RAG
Databases are essential to the operation of RAG systems, as they hold information in a variety of formats which enable the efficient extraction of information. These databases respond when a query is issued by a user. With the RAG system, converting that query into vectors to effectively communicate with and retrieve from the vector database what is required.
The process through which user queries become vector representations epitomizes advanced techniques that allow RAG systems to pull information swiftly and accurately from diverse sources in order to provide answers. Such efficiency proves invaluable, especially where immediacy and accuracy matter greatly.
Thanks to their integration with vector databases, RAG systems can deliver responses that are not only relevant but also reflect the most current updates available.
Integrating Structured and Unstructured Data
Vector databases, which are adept at handling unstructured data like text documents, images and web pages, can be utilized in tandem with SQL databases that manage structured data. By drawing from these diverse sources of information, RAG systems ensure that the generated responses are not only accurate but also richly informed by a broad spectrum of data.
The fusion of structured and unstructured data is a hallmark capability within RAG systems. It allows for an amalgamation where traditional databases containing structured forms meet textual documents brimming with unstructured content. This synthesis permits the crafting of responses carrying greater depth and contextual relevance.
Such integration proves immensely beneficial when tackling tasks steeped in knowledge requirements necessitating an extensive understanding. In merging both forms of data; structured and unrepresented data, the capabilities of RAG AI extend. This is because comprehensive answers become more attuned to context-specific requests.
RAG vs Traditional Search Methods
Conventional search methodologies, such as keyword and semantic searches, frequently do not provide search results that are relevant in a contextual sense. These traditional approaches tend to rely on unchanging techniques, which do not account for the latest updates or feedback from users. On the other hand, RAG systems demonstrate an advanced level of understanding concerning query contexts, markedly improving user experience through delivering more pertinent and sensitive responses.
RAG stands out from traditional methods with its real-time response alteration capabilities using fresh data and insights gained from user interactions. This dynamism guarantees that information is consistently current and applicable—overcoming the drawbacks associated with static searching processes. Vector databases play a pivotal role in RAG by enabling swift retrieval of data arranged as vectors within expansive dimensional spaces.
By harnessing both aspects of retrieval and generation mechanisms, RAG systems deliver a significantly enhanced experience via their search engines when compared to conventional methods. As such tools can yield accurate information promptly, they surpass typical offerings provided by standard search engines or searches in databases.
Applications of RAG in Various Domains
RAG systems demonstrate remarkable flexibility, finding uses in many different sectors. For example, within the financial services industry, RAG is employed to sift through vast amounts of data including transaction logs and compliance-related materials. This aids significantly in refining decision-making procedures. It delivers bespoke financial counsel by combining an individual’s fiscal records with current market dynamics.
When it comes to spotting fraudulence, RAG technologies scrutinize patterns from a multitude of data sources to unearth irregular or dubious transactions, thus strengthening protective measures for banks and shielding customers against scams. In healthcare scenarios, such systems expedite responses to patient inquiries by swiftly gathering pertinent health information which elevates both care quality and client satisfaction.
The realm of customer service also benefits immensely from RAG as chatbots are empowered with real-time data insights allowing them to offer accurate answers tailored specifically to the context at hand—this notably boosts user experience levels. Regarding regulatory adherence concerns. Organizations use these systems not just for tracking changes issued by governance institutions, but also to ensure that they consistently comply with changing directives.
These instances vividly showcase how wide-ranging and profoundly impactful applying RAG technology can be across diverse industries.
Benefits of Using RAG Systems
Vector databases, which house advanced similarity search algorithms, play a pivotal role in RAG systems by bolstering the precision of information retrieval. This enhancement greatly boosts the dependability of these systems and enriches the language model’s functionality through live connections to a broad array of data sources. Generated responses gain added credibility as users can validate answers with source attributions provided alongside AI outputs.
By harnessing RAG architectures, there are significant benefits such as immediate access to up-to-date information from various real-time data sources. As a result, the responses produced are consistently pertinent and fresh. The progressive development in RAG technology is set to focus on enhancing direct interactions with artificial intelligence Augmenting its capacity for efficient two-sided communication.
Lastly, for developers, implementing RAG frameworks provides heightened flexibility when curating data sources, allowing them to tailor content based on evolving user demands accurately. These adjustments ensure that AI remains applicable and highly effective over time. With ongoing improvements expected in continuous learning techniques within these platforms, facilitating uninterrupted updates without substantial retraining periods. Thus establishing RAG systems as an instrumental asset in elevating artificial intelligence accuracy while maintaining relevance and fortifying user confidence.
Challenges in Implementing RAG
Despite the numerous advantages of RAG systems, their implementation is not without challenges. A major issue is the considerable computational and financial costs associated with setting up these systems within high-demand sectors such as finance. RAG can mitigate some of the financial costs by allowing for new data to be added to language models efficiently without necessitating a complete retraining.
Another intricate challenge lies in maintaining data privacy when deploying RAG systems. There’s a potential risk that user information could unintentionally become compromised during model training sessions. To prevent this, it’s essential to establish strong data governance along with strict privacy regulations designed specifically to safeguard personal data. Seren does this with the highest level of security, and avoiding the use of APIs to transfer data.
Lastly, one must consider the ethical dimensions linked to the use of RAG systems carefully. It’s crucial that ongoing research aims at formulating ethical guidelines tailored for these systems which will aid in recognizing and curtailing biases inherent within their retrieval functions and output generation processes. By doing so, we can ensure that our utilization of RAG technology remains just, effective and adheres strictly to principled standards.
Summary
Retrieval Augmented Generation (RAG) improves the functionality of large language models by pulling in information from external, credible sources prior to response generation. This method mitigates typical shortcomings found in large language models such as generating out-of-date or incorrect data, through enabling real-time data retrieval. Consequently, RAG can lower expenses associated with retraining language models and make generative AI more accessible for companies.
By providing users with accurate details along with citations for their origin, RAG boosts confidence among its users. This layer of accountability and dependability positions Retrieval Augmented Generation as a desirable technology for enterprises that are considering the adoption of sophisticated AI tools. It provides developers enhanced influence over the output produced by these language models and facilitates simpler modification to align with changing requirements.
Seren serves businesses by offering an easy and cost-efficient way to incorporate RAG into their operations seamlessly. With Seren’s assistance, businesses can implement Retrieval-Augmented Generation technology to find specific information faster, ultimately saving employees time and boost business productivity. Find out more about Seren today!
Sign up to
Seren today.
Only $32/month.
Recent articles about AI
%20copy.jpg)
What is RAG AI?
Find out how RAG AI integrates business data to enhance AI's response specificity and reliability.

How to Use AI as an Assistant
A practical guide on how to use AI as an assistants can help you with day-to-day.
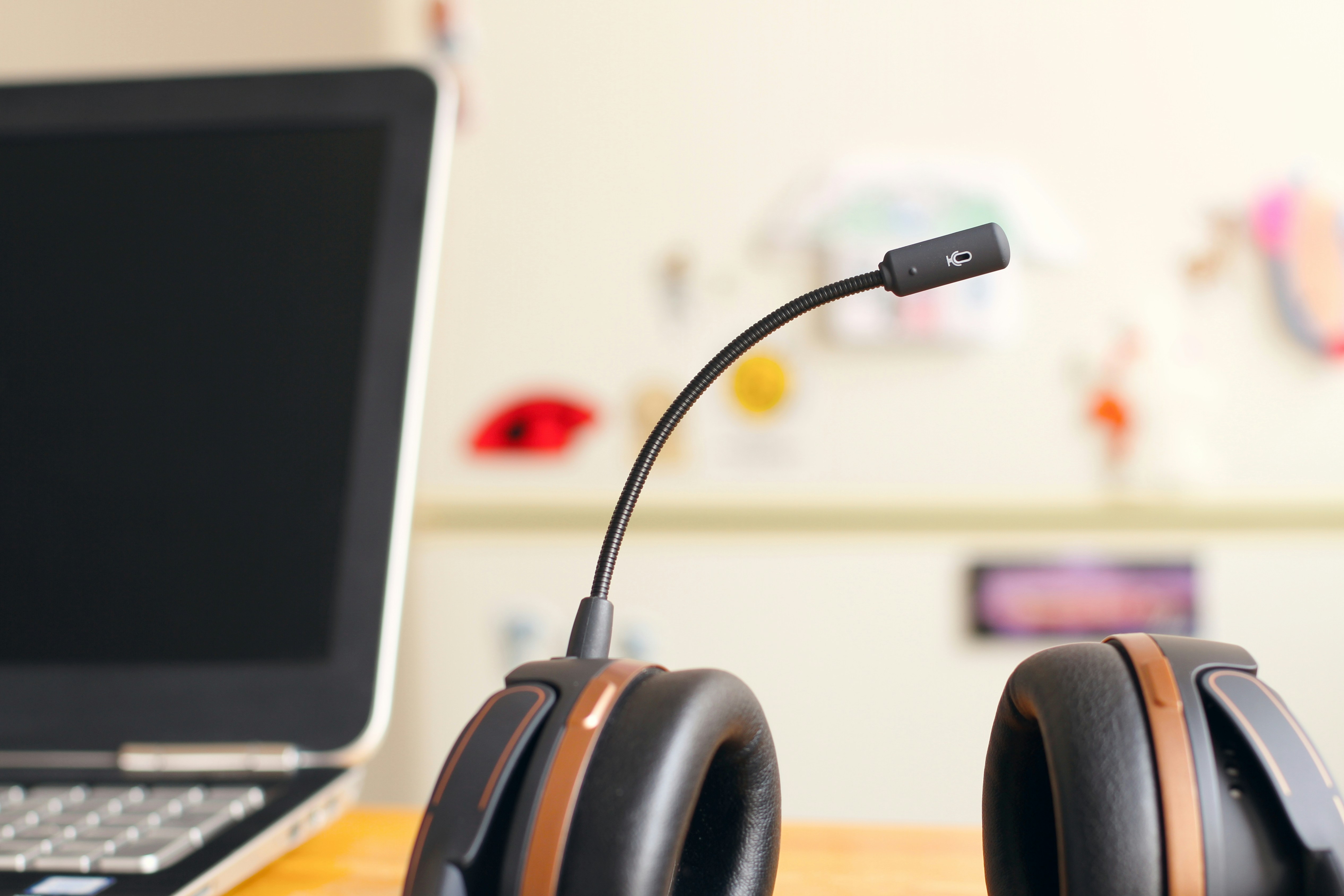
The Top 10 Best AI Assistants
Read our review of the top 10 AI assistants available.
AI that doesn't share your information
Setup a new customizable AI assistant in less then 2 minutes with no AI or developing experience required.